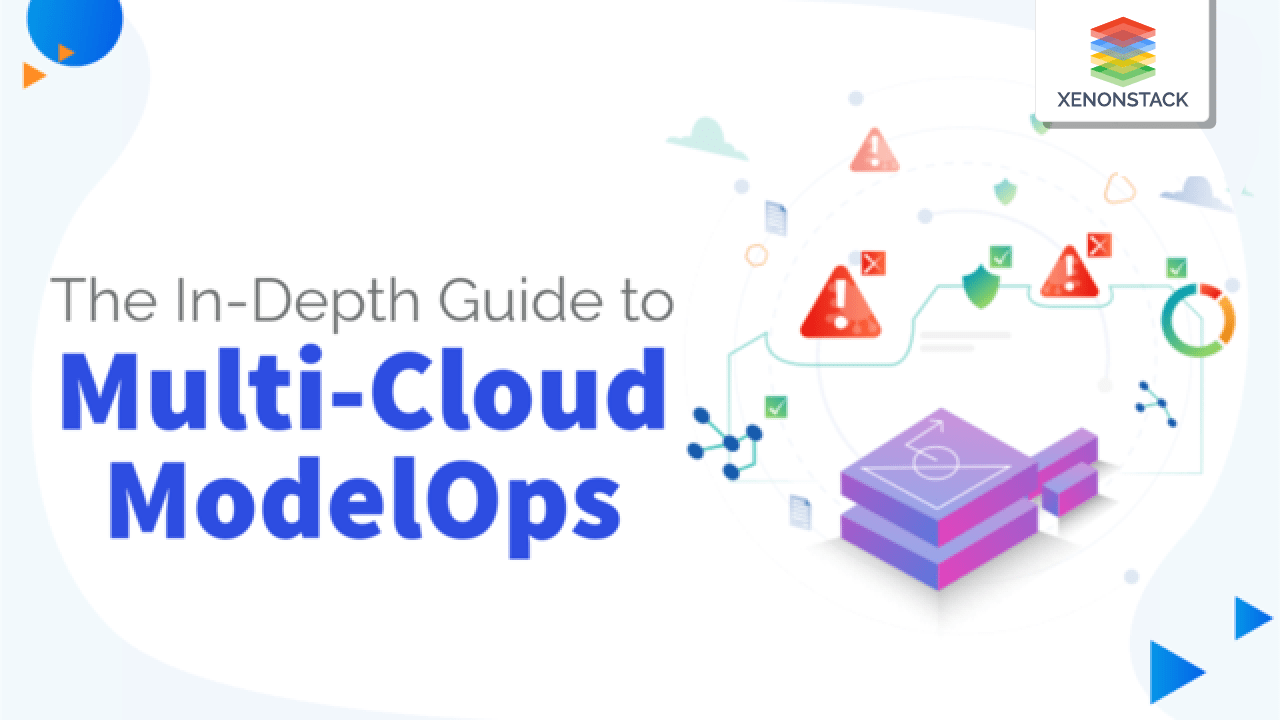
Introduction to Multi-Cloud ModelOps
Businesses need to consistently gather, coordinate and interpret data for AI models and applications to produce value; that's why effective organizations are combining different sets of applications, AI, and analytics development talents with working on a data and AI network that can act as the architecture of information.
What is Multi-Cloud ModelOps?
ModelOps is now the latest approach to the operationalization of models in applications and the synchronization between applications and model pipelines. Using data, tools, and models from edge to core to server, you can maximize data science and AI investments with Multi-cloud ModelOps
Why do we need MultiCloud ModelOps?
MultiCloud ModelOps encompasses the life cycle of maximizing the use of cloud-based models and frameworks that include ML models, optimization models, and other Continuous Integration and Continuous Delivery (CI/CD) integration operating models.
What are the Benefits of Multi-Cloud ModelOps?
- Automate AI lifecycle management: Advance end-to-end production of AI models. By inspiring and reskilling the staff, speed up time to value.
- Speed time to AI outcomes: Link model output to company KPI's. Operationalize the cloud-wide AI model.
- Monitor fairness and mitigate model drift: Explain how models are derived and measure fairness to support the optimal benchmark to correct drift.
- Make AI ready for devops: Selecting the highest performing templates for cloud systems requires only a few minutes.
- Optimize cloud and AI model: Put multi-cloud AI in business to operate. Using versatile consumption models.
- Simplify onboarding: Visual data science and natural language interface forecast and improve performance.
What are the Features of Multi-Cloud ModelOps?
- Generate a Model Pipeline Leaderboard: Data is automatically prepared, the model chosen, feature engineered, and hyperparameter optimized.
- Monitor Machine Learning Models: See potential models and learn how they can be mitigated and how findings can be explained.
- Examine and Debias models: Generate and illustrate the explainability of debiased model points.
- Deploy model Deployment Functions with Applications: Preprocessing of data before sending it to models and handling bugs, including calling several models
- Mix and Match Cloud to Build and Deploy Models: Deploy and virtually drive models everywhere.
- Build, Run, and Manage Models on a Unified Interface: Prepare knowledge, construct templates, and calculate results. Models with feedback loops are improved continuously.
Edge computing is transforming how data from millions of sensors worldwide are treated, stored, and distributed. Click to explore our, Drivers of Edge Computing and Edge AI
Multi-Cloud ModelOps for Applications
Complex, iterative methods are involved in taking AI models from experimentation to development. Access to the training data that complies with privacy, governance, and locality constraints, particularly data moving between various regions, clouds, and regulatory environments, is a significant driver of effective AI investment.
Automating and growing the AI model's lifecycle, including data planning, collecting algorithms, and evaluating and tracking models, will help enterprises produce even better yields. Many organizations that have developed DevOps to deliver applications are now pushing on to construct life cycles for ModelOps that support DevOps. Via the synchronization of ModelOps and DevOps, intelligent automation can further promote agile activity.
Akira AI for Data can maximize cloud and AI investments on a free, extensible architecture operating on any server. Using this tool to develop ModelOps will produce a competitive edge by encouraging businesses to:
- Use natural language interfaces to create predictive timetables, allocations, and schedules, forecast, and maximize market performance.
- Flexible implementation in the chosen area.
- Automate the end-to-end AI lifecycle.
- Empower and reskill developers and professionals in analytics to be AI-ready.
- Speed time to value using sample data, notebooks, and APIs with industry accelerators.
Discover Patterns and Prescribe Actions on a Multi-Cloud Data and AI Platform
What is today's or tomorrow's expected consumer demand? How can we fulfill the demand with equipment, transportation, and staffing? They tuned into observations and forecasts to recommend behavior in a timely, healthy manner to ease decisions. This will enable the organization to:
- Unite the attempt to gather, coordinate and interpret information through every cloud
- To add to data, AI, and application pipelines, widen the talent pool, and reskill employees
- Enable software, innovations, and collaborators' ecosystems to promote exploration and deployment
- Promoting trust and openness through the development of AI model governance as part of their design principles
- To continuously render applications smarter with templates, create, run, and maintain models embedded with DevOps.
Discovery skills essential to help innovators develop new possibilities for growth: connecting, challenging, listening, networking, and exploring.
Accelerating Discovery and Deployment with AI Models on the Data and AI Platform
Akira AI intentionally designed data and AI platforms to satisfy these demands. It automates AI lifecycle management, offers a cohesive experience around the enterprise, expands AI environments, deploys models with a click, and allows models to be automatically monitored for bias, justice, drift, enforcement, and more. Developing and scaling AI with confidence and accountability will help you adapt your company through rapid exploration and implementation.
Uniting DevOps and ModelOps Speeds a Path to Implementing AI into your Applications.
Akira AI lets you scale implementation, have visibility in real-time, and process protection and governance with large data volumes. It allows you to manage your AI models and applications to achieve results that fit the original purpose and intention.
Unified Experience and Governed Frameworks Help Harness the Power of Agile AI Teams.
Akira AI for Data offers a cohesive experience that uses standard patterns and algorithms to direct the development, training, and deployment of models.
Conclusion
A fully integrated multi-cloud data and AI infrastructure can help you simplify AI lifecycles while incorporating hand-offs between technical and operational silos, speeding exploration and execution. A platform also makes AI model consumption simpler and encourages beginners to communicate with experts and learn from them to become main contributors quickly. This is why a data and AI network is the perfect setting for the app and AI cadence synchronization to construct Model Operations (ModelOps) using the Development Operations community (DevOps).
- Click to know What is ModelOps and its Operationalization?
- Read more about Overview of Edge AI Implementation.